I’ve had a baseball visualization book in my head for the better part of a decade but kept setting it aside. Finally, things have come together, and the work has begun. My working title is “Career Arcs: A Visual Analysis of MLB Player Performance”, as the focus will be on the value players have achieved across their playing career.
The initial stage, as is so often the case, is centered on data wrangling, the art of procuring, loading, creating (formulas), analyzing, and finally, visualizing the base data. My process starts with the source data, available under the MIT license, which gives me the ability to use the data however I choose. I will always acknowledge Neil Paine for his great dataset focused on multiple interpretations of WAR (Wins Above Replacement), a widely used metric for baseball statheads. Without this data, creating the book would prove far more challenging.
Exploratoryis one again my primary data wrangling tool; it makes the powerful capabilities of R accessible to a non-coder like myself. In Exploratory, I can load the data, create filters and formulas, and do some pretty cool visualizations. My use is twofold (at least); I can analyze the data on the back end while simultaneously building charts and dashboards for potential use within the book. Here’s an example dashboard I’ve created (in process) where I can see career WAR numbers for any MLB player through the 2024 season:
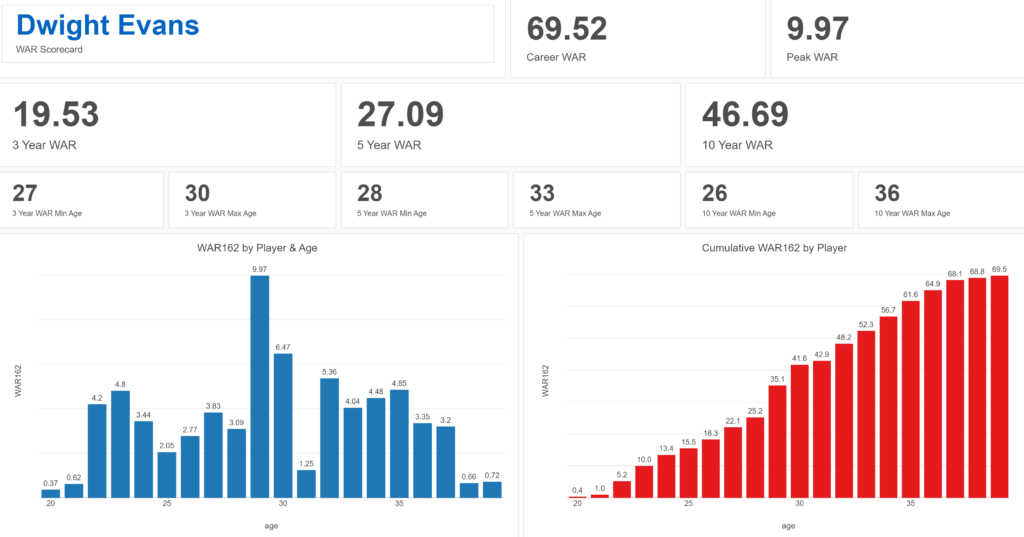
These dashboards allow for data discovery on my end while painting a nice visual picture that may wind up in an appendix section of the book. I love creating charts and dashboards that can be used for more than one purpose!
In addition to working in Exploratory, I am learning the ins and outs of Adobe InDesign, which will be used for page layout, titling, fonts, styles, colors, and any other elements used for book publishing. I have yet to decide how I’ll publish the various versions of the book, other than being fairly certain there will be both e-book and printed versions. Full color printed books can become very expensive to print, so I’m wrestling with a variety of approaches at this stage to maximize readership while also having a print version available at a potentially high price point.
I’ll provide updates as my work progresses, including potential section and chapter content, release dates, and so on. In the meantime, thanks for reading, and let me know your thoughts through my Substack site at Visual Excursions. See you soon!